In the years I worked with lead scoring, Iāve learned that if done right, itās unbeatable and can have an enormous impact on your bottom line. Having worked on the scoring system both for the marketing and sales team in the past, Iāve decided to put together a guide to lead scoring best practices, the ones that proved efficient in practice for me.Ā
I understand that to many people lead scoring could not sound more boring. However, I find it fascinating as it casts a broad web and allows you to work with different departments, from product development to sales. Not to mention the fact that you can enjoy the fruits of your work almost immediately! Plus, if you are a bit of a number and analytics nerd, itās pretty rewarding.
But letās not stall any longer.
Here is my take on lead scoring best practices!
What is Lead Scoring?
Lead scoring is, quite simply, a process of ranking the readiness of your prospects to convert (whether that is to buy or subscribe) by using a numeric system that helps quantify the value of your targets to your company.Ā
The score informs decisions such as how much time and resources to allocate to a lead and increases the speed with which it is processed.Ā
I believe that efficient lead scoring is a critical element for any successful marketing and sales strategy.Ā
In fact, according to DemandGen Report, 41% of participants noticed conversion rate improvement when applying lead scoring. The study also showed that when using lead scoring:
- 53% of participants saw improvement in marketing and sales alignment
- 43% discovered qualified leads that might have been otherwise overlooked
- 43% reported a boost in the number of MQLs (marketing-qualified leads)
- 32% reported an increase in SQLs (sales-qualified leads)
Lead Scoring Best Practices: 13 Tips to Tame
Itās time to get down to it!
Here is my list of lead scoring best practices. Practices that helped me to grow as a marketer and perfect the scoring process.
Design a Lead Scoring Model that Works for YouĀ
A scoring model is a simple act of assigning a numerical value to the key data. The better and more detailed your model, the more accurate your scoring will be. See an example of a scoring chart below:
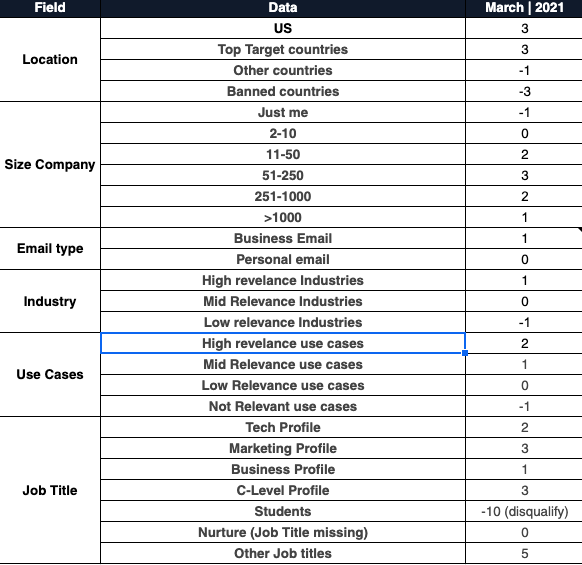
When assigning the numeric values, consider what type of data makes for the ideal customer and the value of the data points in relation to each other. For example, your default setup might be awarding 3 points high relevance industry and 1 to the low one. However, while the industry does play a role, itās the use cases that are a stronger indicator of a good lead so high relevance use cases can be awarded 5 points to represent their weight in the scoring process.
Combine Data Point Types for More Accurate Results
The web is full of articles trying to tell you which data points to score. Though, if you want your scoring system to work, the only place to identify hot leads indicators is your customer base.
In other words, go looking in, not out.Ā
For example, scoring demographic data such as location, company, industry, role, etc., helps you identify the leads which are a good fit for your product or service. However, relying solely on demographic data has its problems as it keeps the scoring quite theoretical. A decision-maker from the right industry and department might still make a terrible lead.Ā Ā
Thatās where behavioral scoring comes in. Behavioral scoring is scoring leads based on actions indicative of interest in your product or service. Some common examples of positive behaviors include:
- Contact requests / contact form submissions
- Email opens, clicks, and forwards
- Social media activity
- Content downloads
- Free trials
- Product demos
Or, if you have a freemium type of product, your behavioral scoring can include user actions inside your application or tool. For example, inside Landbot, subscribers who use certain types of features when building their first bot are more likely to convert. This behavioral data makes up an important part of the scoring strategy.Ā
Collaborate & Align with Sales to Define your Lead Scoring Model
Aligning the marketing and sales team is a critical point.
Sales reps ask the marketing team for more leads, and when marketing provides them, the sales team says that they are of poor quality⦠Sounds familiar?Ā
Likely!Ā
This is, after all, the eternal fight of Marketing vs. The Dark Side (Sales)Ā
Your sales team is closer to your leads than you are. However, if you are offering software as a service, this rings true for your product or customer success teams, as well. This means the sales team (or even product or success teams) is more likely to understand your prospects, their pain points, and triggers much better than you. They are the ones with insights into customer profiles, tendencies, behaviors and so should be the crucial part of creating your scoring model.
For instance, as I already mentioned in the previous point, at Landbot, we use subscriber actions inside the product to help us assess their potential value. Besides demographic data, we look at the speed of bot creation and deployment upon signup as well as the type of features used in the creation process.Ā
Get together with your sales team (and product/customer success team, if relevant) and ask their input on issues such as:Ā
- How many points is each action and piece of demographic data worth?Ā
- Which job titles should be worth the most and which just a little?Ā
- Should attending an online webinar be worth more than downloading an eBook?Ā
- Which in-app actions indicate a higher probability of conversion?
Work on the scoring model together. The collaboration will help both teams understand the work and needs of the other and help improve their relationship in the process.
Incorporate Negative Scores
Not all behavior and actions a prospect takes are positive. The same goes for particular demographic info. They either indicate that the lead is not interested, or they are not a good fit.Ā Ā
Therefore, when creating your score model, make sure to award negative scores for actions or demographic data that decrease the value of a lead. The sales team will be grateful for it as it will save them a great deal of time!
Actions that result in a negative score could include unsubscribing from your email list or visiting your jobs page. You can also assign negatives scores to demographic data such as country or occupation (e.g., if the subscriber indicates they are a student and donāt work for any company, etc.).Ā
Discard Low-Scoring Leads (Set MQL Threshold)
The next step to optimization and time saving is discarding leads with poor qualification.Ā
I know the marketer inside of you begs you to catch as many leads as possible. However, when it comes to leads, quantity is rarely an indicator of success. It can be quite detrimental to your productivity and revenue.Ā
Scoring is supposed to help you identify when a lead becomes sales-ready so they can be sent over to sales as fast and possible so they can engage the prospect while they are still āhot.ā Do not be afraid to leave bad leads behind.Ā
Identify a cut-off threshold for marketing qualified leads.
Depending on your business model, you can have a single threshold or divide the score values into 2-3 tiers. For example, the highest-scoring leads will be passed on to sales, while the ones in the middle tier are put on a self-service sales track.Ā
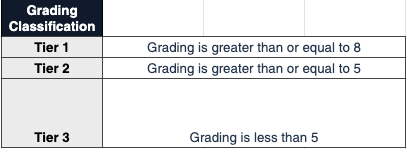
Run a few sample scenarios to help you determine a logical point(s) of qualification. Be sure to revisit it on a regular e basis to ensure that it stays accurate.Ā Ā
Leverage Score Decay
Another method I find quite helpful in identifying worthy leads is score decay.Ā
You see, a lead can tick all the boxes initially but then ends up āghostingā you.Ā
The idea behind score decay is simple, the longer a prospect goes without interacting with your company (missing appointments, not opening emails, not answering calls, etc.), the more points they start to lose.
So, for instance, if a lead hasnāt visited your website or opened an email in two months, you subtract five points from their score. And then more with every passing month they go without engaging.Ā
In combination with negative scores and automatic disqualification, score decay will save the sales team time as they wonāt waste it reaching out to leads that at first glance might appear perfect but are, in fact, unresponsive.Ā
Create Different Paths Based on Lead Scoring
Speaking of tiersā¦
Scoring can do more than just endear you to the sales team.Ā
You can set up your scoring system to segment the leads even further. This way, you can use it to identify their place in the customer journey and set them on a nurture path designed to raise that initial score.Ā
For example, in Landbot, we create different conversational flows based on the score the bot calculates in real-time. Thus, based on a few initial questions, a client who receives a high score is redirected to speak with a live sales agent or given the option to book a meeting. Lower scoring leads are asked a few more questions and then attributedĀ
Establish Clear Lead Scoring Objectives
Among the lead scoring best practices I am recommending, this one is the most straightforward as well as one of the most important ones.Ā
Tracking and measurements are the only ways to know how well you are doing.Ā
If you are scoring your leads, work to establish well-defined objectives for the marketing team.Ā
For example, an objective for your team can be to generate 3000 leads with a score higher than 10 for Qā. A clear goal will help you and your team organize as well as identify issues faster.Ā
Automate Your Scoring Process
Without automation, lead scoring is an absolute nightmare.Ā
To be effective, you need to automate as much of the process as possible.
I prefer to calculate the scores using conversational flows, but thatās hardly surprising. š
Though there are cases in which you can take shortcuts. Some actions are such an obvious indicator of the propensity to buy you can automatically assign them the points necessary to reach the MQL threshold. Take requesting a live product demo, for example! When someone does just that, itās quite obvious sales can get involved.
Ensure your MA and CRM are Integrated
Automation doesnāt end with score calculation. The collected data and the calculated score need to travel to your CRM, preferably instantly.
Your marketing automation (MA) system (be it Landbot or other) performs all the tracking and scoring of prospectsā data and behaviors. You need to ensure the MA communicates with your CRM to export new data there as well as retrieve existing data when interacting with a returning lead.Ā
To create a truly efficient lead scoring mechanism, you need both systems to share the information in real-time. It allows you to react instantly, personalizing the journey for each prospect.Ā
For example, Landbot offers native integration with Salesforce or with any other CRM using the Zapier or webhook block. Thanks to this, when a new lead interacts with a bot, all information is sent to the CRM in real-time.
Automate Alerts to Sales for New MQLs
When a lead becomes qualified, your sales team should be notified as soon as possible.Ā
InsideSales reported that the odds of reaching a new lead decrease over 10x if you take over an hour from the moment of the prospect first showing interest. Also, the odds of [sales] qualifying that lead drop 6x after the first hour.
Setting up instant automatic notifications is the best way to ensure quick outreach once a lead is marketing qualified. The notification should be part of your automated lead scoring workflow.Ā
For me, notifications are just another reason why I enjoy working with lead-scoring landbots.Ā
Adding a Slack or email notification inside the flow takes a second. Plus, when scoring occurs in the middle of a conversation, sales agents can jump right in and turn the automated chat into a live one right after receiving a notification!Ā
Talk about quick response time!
Track MQL to Conversion Rates
After you qualify a lead and pass them to sales and the rep opens a conversation turning it into a sales accepted lead (SAL). SAL, Quite simply, it means the sales team accepted them as valid and begin working with them. If all goes well, that conversation opens an opportunity with that lead, turning it into a sales-qualified lead (SQL).
To ensure the quality of your lead scoring process, you should measure the percentage of your MQLs that actually turn into SQLs. Itās the best indicator you are sending the sales department leads worth their time and effort. If the conversion percentage is low then you need to go back and revisit your scoring process. The issue might be with the scoring itself or simply with the selected threshold.
Continuously Review your Lead Scoring System
Creating a scoring model is a process that never ends.Ā
The scoring and conversion of each parameter need continual revisions and adjustments. Review the measurements regularly and make adjustments.Ā
Do not underestimate this step! Itās a sure way to boost the marketing teamās performance and collect key data to create a much clearer go-to-market strategy as well as keep the ideal customer profile (ICP) up to date.Ā
Revisit the scoring process monthly or once per quarter⦠whatever pace fits your workflow - but never forget about it!
See an example below:
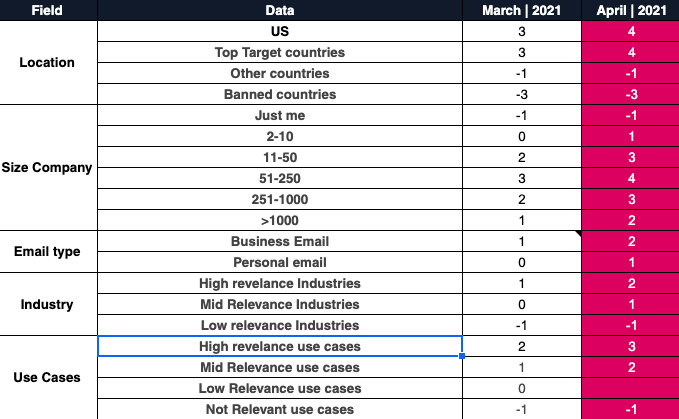
Donāt forget to bring your sales team and other relevant departments to the table when the revisions happen. They are a vault of valuable feedback on how the lead scoring mechanism is working and can help you identify the areas needing improvement.
3 Ways to Build a Lead Scoring Model inside your Conversational Flow
Lead scoring automation can be done in a number of ways, but my favorite, by far, is chatbots.Ā
I understand that my opinion might appear quite biased with me being a Landbotter through and through. However, Iāve worked with lead scoring long enough to try it all, and there are definite advantages to using conversational interfaces to do the scoring.Ā
Why?
Firstly, it makes the information submission more natural and fun.Ā
Secondly, bot builders such as Landbot offer features to get the scoring done inside the bot in real-time.Ā
Here is a sneak peek into the three most used scoring methods in Landbot.Ā
1. Method #1: Condition + Set Variable
This Landbot lead scoring method is the simplest to understand at first glance is easier. It uses the āConditional Logicā and āSet a Variableā blocks to calculate the score stored under the previously created @score variable:

The operation is as follows:Ā
- Using the conditions block to determine if the prospects use case response equals "Lead Generation";
- If yes, use the green output to add the points corresponding to this use case (+2 in this case) and take the user to the next question;
- If not, repeat the same condition with another answer option (in this case, the Customer Support use case) and repeat the process, assigning score addition using the green output and the red output to score the next use case option.
The process is simple but can get a bit messy inside the builder, especially if you are trying to build a more complex scoring process. It makes it difficult to review:Ā
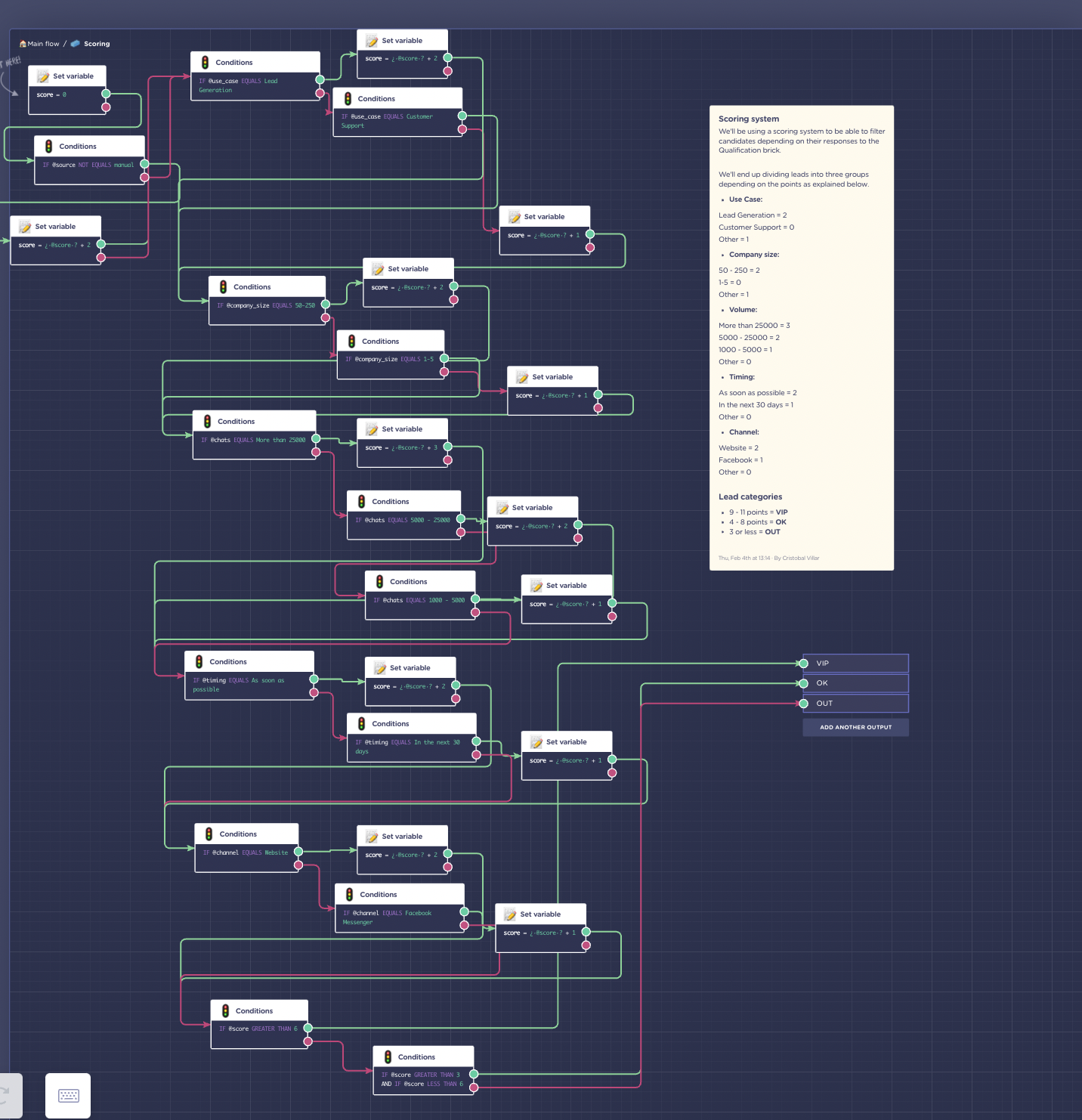
Still, if you need a simpler scoring mechanism, itās the quickest and easiest way.Ā
If interested, check out the full tutorial for the conditional logic scoring method.Ā
For more complex scoring needs, Landbot created the "Lead scoring" block that solves the āmessinessā problem.
2. Method #2: Lead Score Block
This block was quite literally created as an evolution of the first method, and itās very easy to use!
Just check out the video below to learn more about it:
3. Method #3: Formulas
Last but not least, there is scoring using the Formulas block - the most badass of all options.Ā
Formulas are a no-code way to implement usually code-requiring calculations and actions.Ā
Think of it as a sophisticated version of formulas you know from tools like Google sheets.Ā
I admit, thereās a bit of a learning curve with Formulas, but the benefits are worth it. It allows you to create scoring bots with nearly magical abilities. Well, maybe thatās an exaggeration but using Formulas for lead scoring is a game-changer for any marketer.Ā
I know it was for me!ā
I explain the process in this 'Formulas for Lead Scoring Tutorial.' šāāā